Many investors agree: Active strategies that try to beat the stock market have periods where they perform well (contributing to outperformance) and periods where they perform poorly (contributing to underperformance.)
In other words, any strategy that tries to beat the stock market – even the ones that “really work” – will have “volatility.” Even a strategy that “works” on average in the long run, will have some years of great outperformance, some years of disappointing underperformance, and some years of what we’d expect – some outperformance.
“Quant” factor strategies, which seek to objectively target market-beating sources of return with strong statistical support from academic research, also have volatility – some great years, some bad years, some average years. Some notable factor strategies are:
- Market Beta: Also known as “passive indexing,” this strategy seeks to earn the returns generated by the stock market.
- Value: Undervalued stocks tend to outperform expensive ones in the long run.
- Momentum: Stocks whose prices have lately been rising tend to outperform stocks whose prices have lately been falling.
- Profitability: Stocks of more profitable companies tend to outperform stocks of minimally or unprofitable companies.
Stock Market & Long-Short Factor Strategy Returns, 2000 – 2023
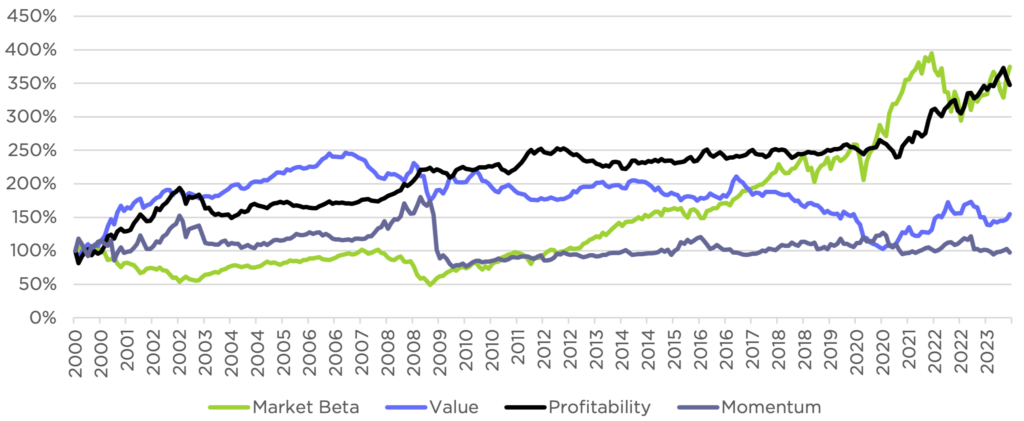
Stock Market & Long-Short Factor Strategy Returns, 1963 – 2023
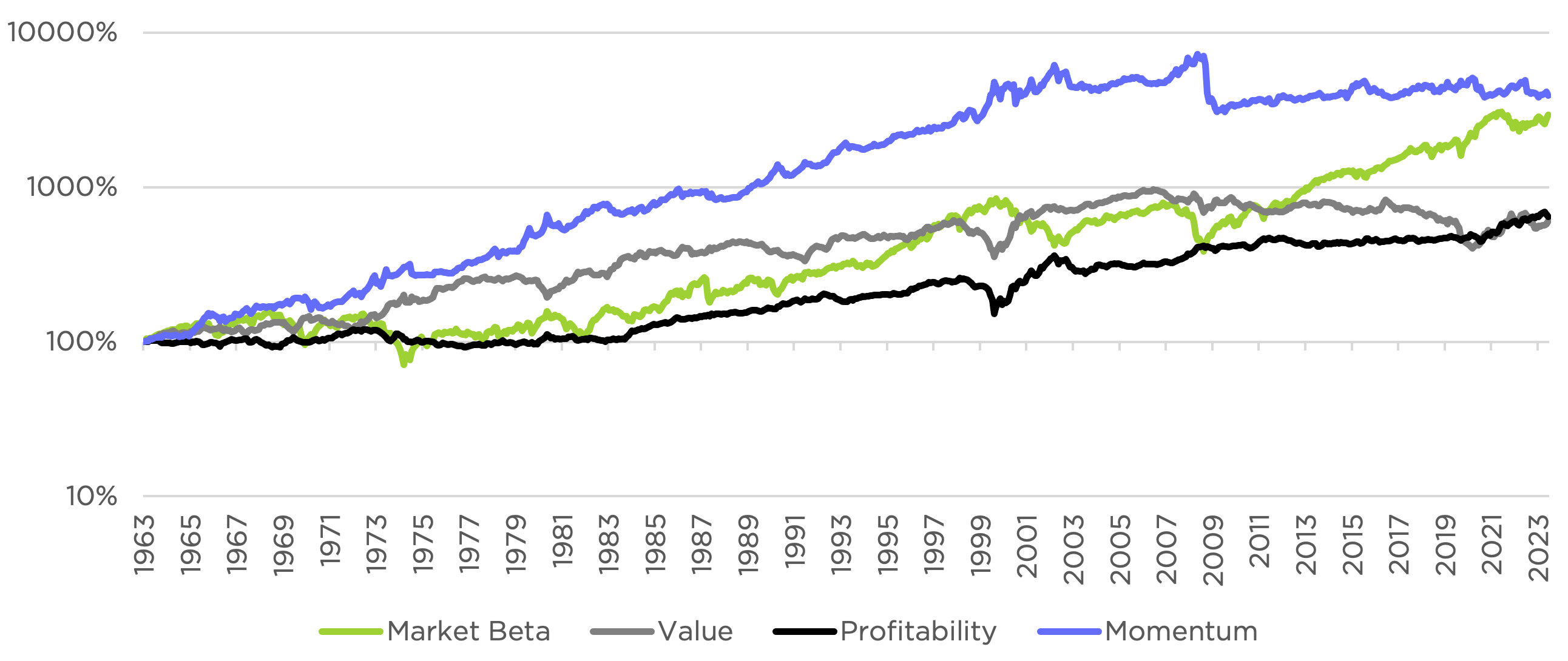
Volatility of active factor strategies is evident from history. This table shows the average, best year, worst year, and standard deviation (a numerical measure of volatility) for each factor strategy. The three factor strategies represent long-short “market neutral” strategies that buy the stocks with the best ratings for each factor and sell short the stocks with the worst ratings.
Average Quarterly Performance During Periods of Rising, Falling & Stable Rates
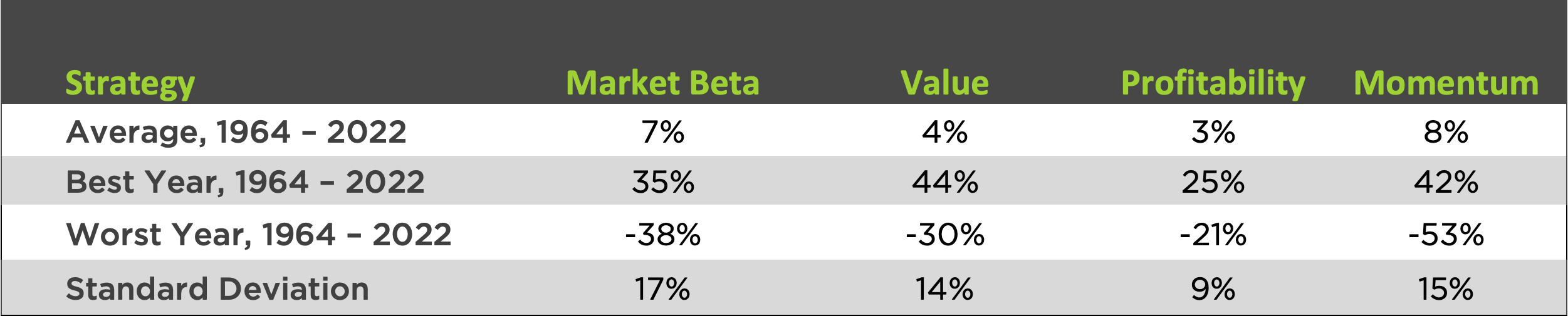
We see the pattern here. Each strategy has positive long-run expectation (the average return to each strategy is positive since the 1960s); some really outstanding years (20+ percentage points of positive performance) and some really tough years (20+ percentage points of negative performance). Each strategy has volatility. Investors can benefit to the extent they can earn the returns to these strategies while managing the volatility.
Conclusion
One way to manage volatility is to diversify factor strategies. Rather than target only value, only momentum, or only profitability, an investor can help reduce volatility by investing in strategies that target multiple factors at the same time. Counterpoint uses this technique across its factor-oriented strategies.
Another way to manage volatility is to use machine learning models to identify factors that have attractive prospects. These models incorporate a lot of data to detect historical patterns in factor strategies’ responses to different investing environments and seek to exploit those patterns when positioning equity portfolios. Counterpoint’s funds make extensive use of machine learning to optimize factor exposures within its equity products on an ongoing basis.
Active management strategies have volatility, which can contribute to outperformance and underperformance. When seeking differentiated sources of return in a long-only or a long-short context, investors need strategies to manage that volatility and give them a good chance of realizing their long-run return potential. Machine learning processes offer a novel way to identify and manage risks associated with factor investing strategies beyond traditional diversification among various strategies that seek to beat the market.